Linking online and offline social networks to better predict real world impact
Prof. Lei Ying leads a new Multidisciplinary University Research Initiatives (MURI) project that seeks to develop nonlinear, multi-scale, multi-network models to understand how the interplay between online networks (such as social media and other online communities) and offline networks can impact real world behaviors and events.
“We want to apply machine learning, data science, and AI tools—which have revolutionized computer science—to the social sciences,” Ying said. “Having new mathematical models and machine learning tools to understand how online and offline networks impact each other, we can better predict disruptive human behaviors .”
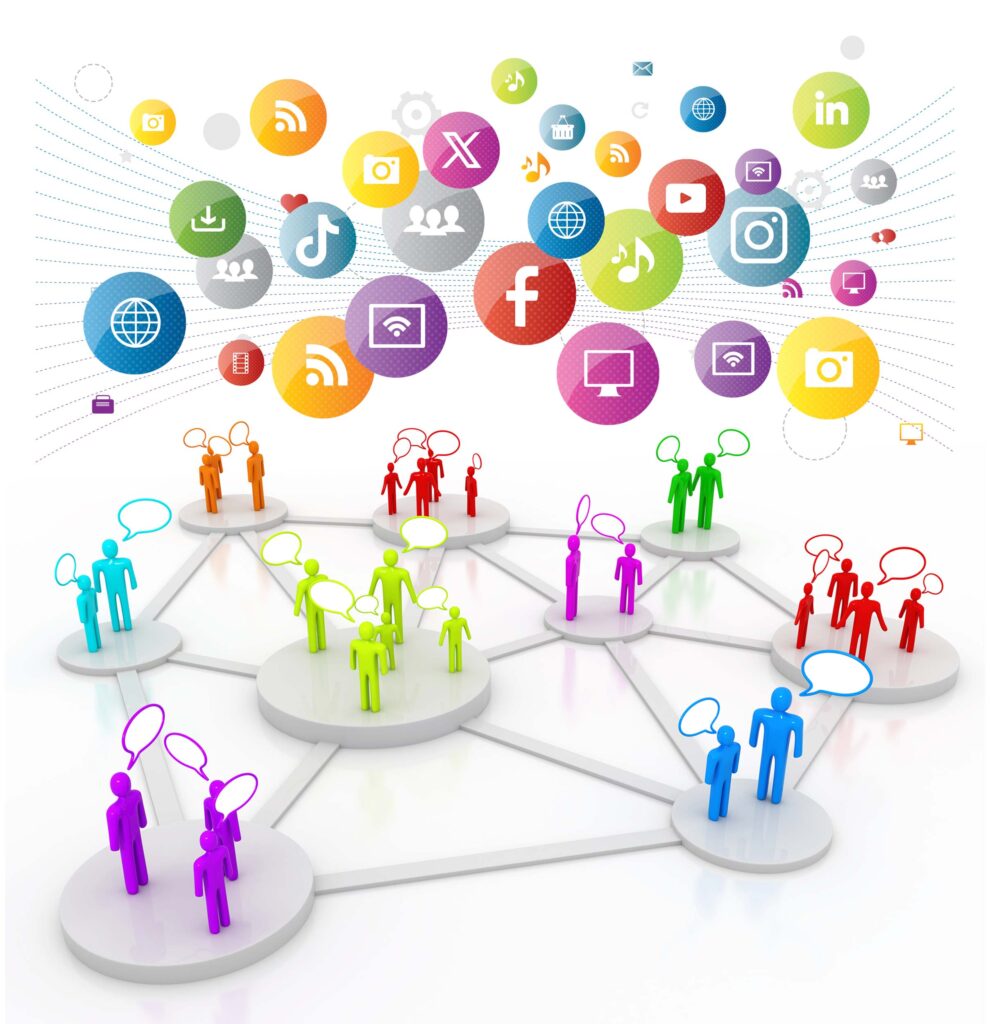
Until recently, the study of human social networks has largely been segregated by type: offline in person networks vs online social communities. However, understanding the interaction and influence between these types of networks could greatly help social scientists predict events that lead to unrest, including violence and political upheaval.
“By understanding how online networks impact offline behavior and vice versa, we can develop better interventions,” Ying said. “For instance, what’s the best solution when false information is spreading? Is it better to provide truthful information or to try and limit the spread of the misinformation? What kinds of policies would be most effective?”
While there has been much attention to the spread of conspiracy theories and the normalization of politically extreme opinions online, there are no robust mathematical models and analytical tools to explain how this might impact real world events. This has led to inaccurate assumptions about the role online communities play in offline movements.
For example, many assume that online activity triggers real world activism events, but this has yet to be proven, and existing research suggests the connection is more complicated. For instance, studies on the Arab Spring showed social media use to be the result of offline attitudes and activities, rather than the cause.
“Online networks may not be the origin for real world behavior, but because they can spread information so fast, they can have a big impact on how these events play out,” Ying said. “The event probably would have happened regardless, but online networks mean the event could be on a much bigger scale, and therefore a lot more disruptive.”
To develop a mathematically robust model that captures the interplay between online and offline networks, Ying’s team needs to address four major challenges:
- Online and offline network alignment: Ideally, people’s online networks would closely reflect their offline networks, which would make it easier to track and identify the interactions. However, some people, particularly those embedded in extremist online groups, may have very different online and offline networks, and they may even hide their association with certain online groups.
- The development of sparse low dimensional representations both for online and offline networks: Cyber and physical domains are very different, and operate at different spatial and temporal scales. However, they may share common low-dimensional representations that reflect the fundamental structure information of the networks.
- Understanding the mechanism of opinion dynamics: How do dynamics in one domain, such as opinion dynamics in offline communities, then propagate to online communities?
- Predicting the cascade trigger: Identifying the threshold where the interplay between online and offline networks would then result in either information going viral or triggering real world behavior.
The three-year MURI is called “Understanding Social Network-Transcendent Online/Offline Behavioral Dynamics: From Data to Models to Prediction.” It is funded by the Air Force Office of Scientific Research. Ying is collaborating with co-PIs Rayadurgam Srikant and Hanghang Tong from the University of Illinois at Urbana-Champaign, and Naomi Leonard from Princeton University.